top of page
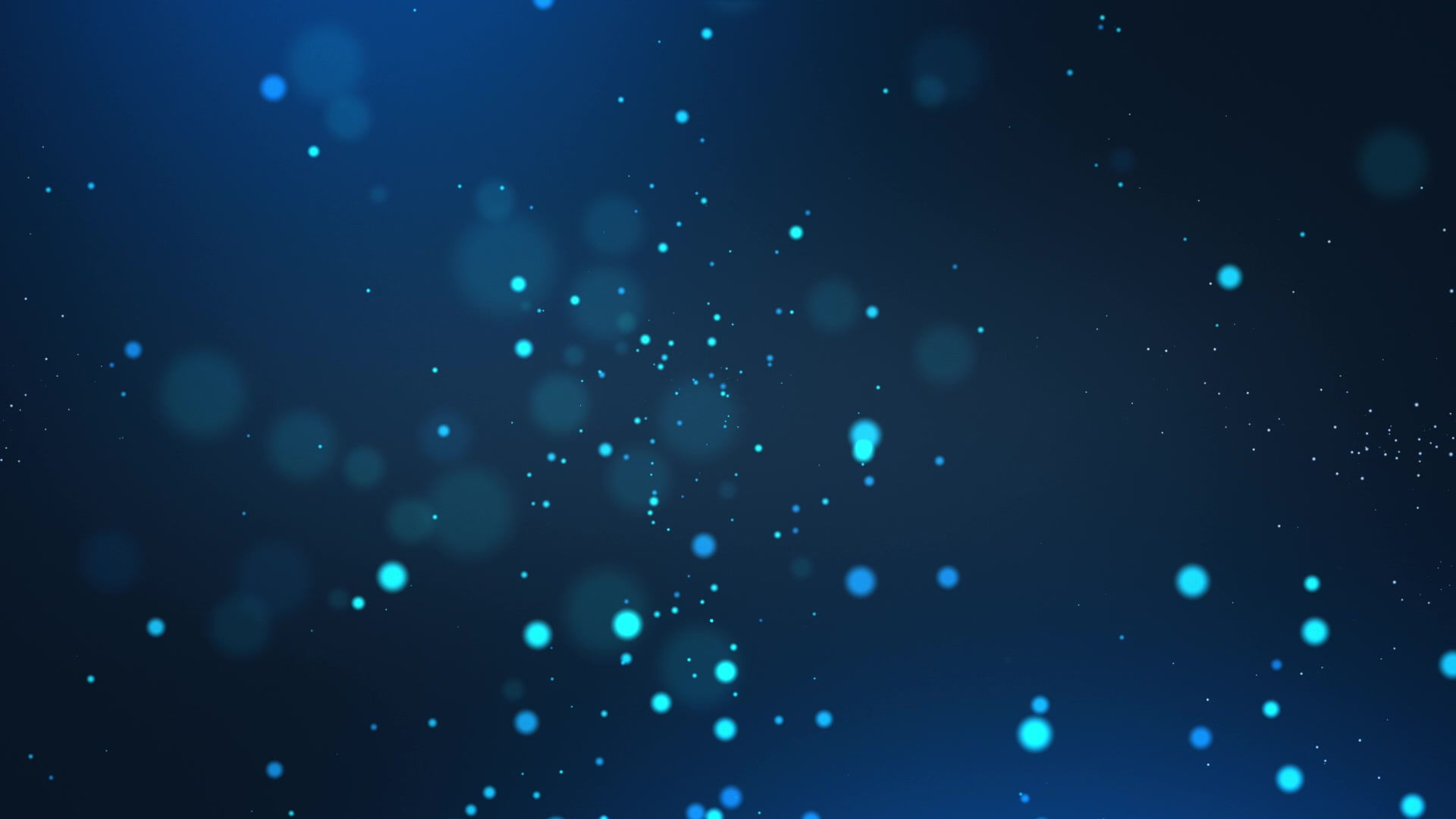
Futuristic Greedy approach to HS Unmixing (IEEE TGRS 2014)
Spectra measured at a single pixel of a remotely sensed hyperspectral image is usually a mixture of multiple spectral signatures (endmembers) corresponding to different materials on the ground. Sparse unmixing assumes that a mixed pixel is a sparse linear combination of different spectra already available in a spectral library. It uses sparse approximation techniques to solve the hyperspectral unmixing problem. Among these techniques, greedy algorithms suite well to sparse unmixing. However, their accuracy is immensely compromised by the high correlation of the spectra of different materials. This work proposes a novel greedy algorithm, called OMP-Star, that shows robustness against the high correlation of spectral signatures. We preprocess the signals with spectral derivatives before they are used by the algorithm. To approximate the mixed pixel spectra, the algorithm employs a futuristic greedy approach that, if necessary, considers its future iterations before identifying an endmember. We also extend OMP-Star to exploit the non-negativity of spectral mixing. Experiments on simulated and real hyperspectral data show that the proposed algorithms outperform the state of the art greedy algorithms. Moreover, the proposed approach achieves results comparable to convex relaxation based sparse approximation techniques, while maintaining the advantages of greedy approaches.

We propose a constrained matrix factorization approach for linear unmixing of hyperspectral data. Our approach factorizes a hyperspectral cube into its constituent endmembers and their fractional abundances such that the endmembers are sparse nonnegative linear combinations of the observed spectra themselves. The association between the extracted endmembers and the observed spectra is explicitly noted for physical interpretability. To ensure reliable unmixing, we make the matrix factorization procedure robust to outliers in the observed spectra. Our approach simultaneously computes the endmembers and their abundances in an efficient and unsupervised manner. The extracted endmembers are nonnegative quantities, whereas their abundances additionally follow the sum-to-one constraint. We thoroughly evaluate our approach using synthetic data with white and correlated noise as well as real hyperspectral data. Experimental results establish the effectiveness of our approach.

bottom of page